Using Artificial Intelligence Applications and Technologies to Reduce Road Congestion Rates in Arab Countries
Authors
Keywords:
Security Studies, Artificial Intelligence, Traffic and transportation, Road CongestionSynopsis
With the emergence of Artificial Intelligence (AI) and the growing expectations of the role its emerging technologies can play in effectively tackling transportation system challenges, the integration of AI technologies into urban transportation systems has garnered increased attention. This is due to their capabilities to process and analyze vast amounts of data in real-time and the anticipated potential of AI as a promising means to tackle traffic congestion and improve vehicle routing in urban areas. By harnessing the power of AI, it becomes possible to develop smart systems that dynamically adapt to traffic conditions, thereby enhancing transportation efficiency, reducing congestion-related issues, and promoting sustainable urban development.
Accordingly, as part of the efforts of Naif Arab University for Security Sciences, this report aims to explore the potential of employing AI techniques to reduce congestion rates on roads in Arab cities. The report delves into the intersections between AI and urban transportation, with a particular focus on improving vehicle routing and alleviating traffic congestion in the Arab region. The primary objective is to uncover the most important AI techniques and algorithms related to the field of transportation and the opportunities for their application and utilization in designing transportation policies for the Arab region that reduce congestion rates, shorten travel times, and contribute to road safety. This report is based on a systematic review of published research literature on papers related to the problem of traffic congestion and AI techniques used in measures to reduce it.
أولًا: المراجع العربية
برنامج موائل (2022). المكتب الإقليمي للدول العربية - لمحة عامة عن عام 2022. الأمم المتحدة.
ثانيًا: المراجع الأجنبية
Abduljabbar, R., Dia, H., Liyanage, S. and Bagloee, S, A. (2019). Applications of artificial intelligence in transport: An overview. Sustainability. 11: 189; doi:10.3390/su11010189.
Aeberhard, M., Rauch, S., Bahram, M., Tanzmeister, G., Thomas, J., Pilat, Y., Homm, F., Huber, W. and Kaempchen, N. (2015). Experience, results and lessons learned from automated driving on Germany’s highways. IEEE Intell. Transp. Syst. Mag. 7: 42–57.
Aftabuzzaman, M. (2007). Measuring traffic congestion—A critical review. In Proceedings of the 30th Australasian transport research forum (ATRF), Melbourne, Australia.
Anthony, D. (2004). Still Stuck in Traffic: Coping with Peak-Hour Traffic Congestion. Brookings Institution Press Washington, DC.
Azgomi, H.F. and Jamshidi, M. (2018). A brief survey on smart community and smart transportation. In Proceedings of the 2018 IEEE 30th International Conference on Tools with Artificial Intelligence (ICTAI), Volos, Greece, 5–7 November 2018; pp. 932–939.
Biswas, A. and Wang, H. C. (2023). Autonomous vehicles enabled by the integration of IoT, Edge Intelligence, 5G, and Blockchain. Sensors. 23:1963. Available at: https://doi.org/10.3390/ s23041963
BPY, L. and Huang Z. (2022). Spatio-temporal variations of traffic congestion under work from home (WFH) arrangements: Lessons learned from COVID-19. Cities. 2022 May; 124:103610.
Burton, K.J. (2010). Transport economics. Elgar. ed. 3rd.
Chen, Q., & Wang, W. (2019, December). Multi-modal neural network for traffic event detection. In 2019 IEEE 2nd International Conference on Electronics and Communication Engineering (ICECE) (pp. 26-30). IEEE.
Conde, M. L., & Twinn, I. How Artificial Intelligence is Making Transport Safer, Cleaner, More Reliable and Efficient in Emerging Markets. 2019.
Datta, S.K., Da Costa, R.P.F., Härri, J. and Bonnet, C. (2016). Integrating connected vehicles in internet of things ecosystems: Challenges and solutions. In Proceedings of the 2016 IEEE 17th International Symposium on a World of Wireless, Mobile and Multimedia Networks (WoWMoM), Coimbra, Portugal, 21–24 June 2016, pp. 1–6.
de Souza, A. M., & Villas, L. A. (2015). A new solution based on inter-vehicle communication to reduce traffic jam in highway environment. IEEE Latin America Transactions, 13(3), 721-726.
Dikshit, S., Atiq, A., Shahid, M., Dwivedi, V., & Thusu, A. (2023). The Use of Artificial Intelligence to Optimize the Routing of Vehicles and Reduce Traffic Congestion in Urban Areas. EAI Endorsed Transactions on Energy Web, 10.
Djahel, S., Doolan, R. and Muntean G.M. (2015). A communications-oriented perspective on traffic management systems for smart cities: challenges and innovative approaches. IEEE Commun. Surv. Tutor. 17(1): 125–151.
DoT. (2015). Traffic congestion and reliability: trends and advanced strategies for congestion mitigation. Available at:
ESCWA. (2019). SDG 7 IN ARAB REGION. policy Brief. No. 10United Nations.
European Conference of Ministers of Transport. (2007). Cutting transport CO2 emissions: What progress?
Falcocchio, J.C. and Levinson, H.S. (2015). Road traffic congestion: a concise guide (Springer International Publishing AG.
Fattah, M. A., Morshed, S. R. and Kafy, A. A. (2022). Insights into the socio-economic impacts of traffic congestion in the port and industrial areas of Chittagong city, Bangladesh. Transportation Engineering. 9: 100-122.
Guerrero-Ibanez, J.A., Zeadally, S. and Contreras-Castillo, J. (2015). Integration challenges of intelligent transportation systems with connected vehicles, cloud computing, and internet of things technologies. IEEE Wirel. Commun. 22:122–128.
Gomathi, B., Ashwin, G. (2022). Intelligent Traffic Management System Using YOLO Machine Learning Model. In: Peter, J.D., Fernandes, S.L., Alavi, A.H. (eds) Disruptive Technologies for Big Data and Cloud Applications. Lecture Notes in Electrical Engineering, vol 905. Springer, Singapore.
Haque, A.B., Bhushan, B. and Dhiman, G. (2022). Conceptualizing smart city applications: Requirements, architecture, security issues, and emerging trends. Expert Syst. 39: e12753.
Health Effect Institute (HEI) Panel on The Health Effects of Traffic-Related Air Pollution (2010). Traffic-Related Air Pollution: A Critical Review of the Literature on Emissions, Exposure and Health Effects. HEI Special Report 17. Boston, MA: Health Effect Institute.
Herath, H.M.K. and Mittal, M. (2022). Adoption of artificial intelligence in smart cities: A comprehensive review. International Journal of Information Management Data Insights. 2 (1):100076. Available at: HTTPs://doi.org/10.1016/j.jjimei.2022.100076. http://www.ops.fhwa.dot.gov/congestion_report/chapter2.htm
Huang, X., Yang, X., Chang, Y. (2007). Causes of urban traffic congestion. Journal of Transportation Engineering and Information. 5: 108-113.
Hussain, R. and Zeadally, S. (2018). Autonomous cars: Research results, issues, and future challenges. IEEE Commun. Surv. Tutor. 21:1275–1313.
Iyer, L.S. (2021).AI enabled applications towards intelligent transportation. Transportation Engineering. 5:100083 (ISSN 2666-691X). Available at: https://www.sciencedirect.com/science/article/pii/S2666691X21000397 .
Karouani, Y., Elhoussaine, Z. (2018). Toward an Intelligent Traffic Management Based on Big Data for Smart City. In: Ben Ahmed, M., Boudhir, A. (eds) Innovations in Smart Cities and Applications. SCAMS 2017. Lecture Notes in Networks and Systems, vol 37. Springer, Cham.
Kaysi, I., & Chaaban, F. B. (2013). Transitioning to the green economy: the Arab transport sector policy brief. International Journal of Sustainable Development and Planning, 8(3), 305-320.
Kim, D., & Jeong, O. (2019). Cooperative traffic signal control with traffic flow prediction in multi-intersection. Sensors, 20(1), 137.
Kong, X., Xu, Z., Shen, G., Wang, J., Yang, Q., & Zhang, B. (2016). Urban traffic congestion estimation and prediction based on floating car trajectory data. Future Generation Computer Systems, 61, 97-107.
Li, Y. (2021). IF-MABAC method for evaluating the intelligent transportation system with intuitionistic fuzzy information. Journal of Mathematics. Volume 2021 | Article ID 5536751 > Available at: https://doi.org/10.1155/2021/5536751
Lin, C., Han, G., Du, J., Xu, T., Shu, L. and Lv, Z. (2020). Spatiotemporal congestion-aware path planning toward intelligent transportation systems in software-defined smart city IoT. IEEE Internet Things J. 7: 8012–8024.
Litman, T. (2007). Congestion reduction strategies: Identifying and evaluating strategies to reduce traffic congestion; Victoria Transport Policy Institute: Victoria, BC, Canada.
Lopez Conde, M., & Twinn, I. (2019). How artificial intelligence is making transport safer, cleaner, more reliable and efficient in emerging markets.
Mandal, V., Mussah, A. R., Jin, P., & Adu-Gyamfi, Y. (2020). Artificial intelligence-enabled traffic monitoring system. Sustainability, 12(21), 9177.
McGregor, R.V., Eng, P. and MacIver, A. (2003). Regional its architectures—From policy to project implementation. In Proceedings of the Transportation Factor 2003. Annual Conference and Exhibition of the Transportation Association of Canada. (Congres et Exposition Annuels de l’Association des transport du Canada) Transportation Association of Canada (TAC), St. John’s, NL, Canada.
Ministry of Land, Infrastructure, Transport and Tourism 2018: Roads in Japan. MLIT. Tokyo, Japan.
Mohammadi, M. and Al-Fuqaha, A. (2018). Enabling cognitive smart cities using big data and machine learning: Approaches and challenges. IEEE Commun. Mag. 56: 94–101.
Mussone, L., Grant-Muller, S. and Laird, J. (2015). Case Stud. Transp. Policy. 3: 44–54.
Nasim, R. and Kassler, A. (2012). Distributed architectures for intelligent transport systems: A survey. In Proceedings of the 2012 Second Symposium on Network Cloud Computing and Applications, London, UK 3–4 December 2012. pp. 130–136.
Nigam, N., Singh, D.P. and Choudhary, I. (2023). A review of different components of the intelligent traffic management system (ITMS). Symmetry, 15, 583. https:// doi.org/10.3390/sym15030583.
Nikitas, A., Michalakopoulou, K., Njoya, E., T. and Karampatzakis, D. (2020). Artificial intelligence, transport, and the smart city: definitions and dimensions of a new mobility era. Sustainability.12: 2789; doi:10.3390/su12072789.
Oladimeji, D., Gupta, K., Kose, N.A., Gundogan, K., Ge, L. and Liang, F. (2023). Smart Transportation: An overview of technologies and applications. Sensors. 23: 3880. Available at: https://doi.org/10.3390/ s23083880.
Olugbade, S., Ojo, S., Imoize, A. L., Isabona, J., & Alaba, M. O. (2022). A review of artificial intelligence and machine learning for incident detectors in road transport systems. Mathematical and Computational Applications, 27(5), 77.
Ouallane, A. A., Bahnasse, A., Bakali, A., & Talea, M. (2022). Overview of road traffic management solutions based on IoT and AI. Procedia Computer Science, 198, 518-523.
Pan, J., Popa, I.S., Borcea, C. (2016). Divert: a distributed vehicular traffic re-routing system for congestion avoidance. IEEE T Mobile Comput. PP (99): 1.
Pop, M.D., Pandey, J. and Ramasamy, V. (2020). Future networks 2030: Challenges in intelligent transportation systems. In Proceedings of the 2020 8th International Conference on Reliability, Infocom Technologies and Optimization (Trends and Future Directions) (ICRITO), Noida, India, 4–5 June 2020, pp. 898–902.
Reed, T. and Kidd, J. (2019). Global traffic scorecard; INRIX Research: Altrincham, UK.
Rocha Filho, G.P., Meneguette, R.I., Neto, J.R.T., Valejo, A., Weigang, L., Ueyama, J., Pessin G. and Villas, L.A. (2020). Enhancing intelligence in traffic management systems to aid in vehicle traffic congestion problems in smart cities. Ad Hoc Netw. 107:102265.
Sarker, I.H., Kayes, A. and Watters, P. (2019). Effectiveness analysis of machine learning classification models for predicting personalized context-aware smartphone usage. J. Big Data. 6:1–28.
Seo, S. B., & Singh, D. (2018, November). Smart Town Traffic Management System Using LoRa and Machine Learning Mechanism. ReSENSE Lab, Hankuk University of Foreign Studies, Global Campus – Yongin, South Korea.
Shukla, S., Balachandran, K. and Sumitha, V. (2016). A framework for smart transportation using Big Data. In Proceedings of the 2016 International Conference on ICT in Business Industry & Government (ICTBIG), Indore, India, 18–19 November 2016, pp. 1–3.
Sriratnasari, S.R., Wang, G., Kaburuan, E.R. and Jayadi, R. (2019). Integrated smart transportation using IoT at DKI Jakarta. In Proceedings of the 2019 International Conference on Information Management and Technology (ICIMTech), Bali, Denpasar, 19–20 August 2019. Volume 1, pp. 531–536.
Statista. (2023). MENA Road Traffic Delay by City. Retrieved March 4, 2023, from https://www.statista.com/statistics/1331996/mena-road-traffic-delay-by-city/#statisticContainer
Stipancic, J., Miranda-Moreno, L. and Saunier, N. (2017). Impact of congestion and traffic flow on the crash frequency and severity: application of smartphone-collected GPS travel data. Transportation Research Record. 2659(1): 43-54.
Systematics, C. (2005). Traffic congestion and reliability: Trends and advanced strategies for congestion mitigation; Cambridge Systematics Inc.: Cambridge, MA, USA.
Tang, C., Hu, W., Hu, S. and Stettler, M.E.J. (2020). Urban traffic route guidance method with high adaptive learning ability under diverse traffic scenarios. IEEE Trans. Intell. Transp. Syst.22:2956–2968.
Umesh, M. (2022). Improving India’s traffic management using intelligent transportation systems. Electronic Theses, Projects, and Dissertations. Available at: https://scholarworks.lib.csusb.edu/etd/1548.
United Nations, (2023). The State of Arab Cities Report 2022. United Nations Publications.
Wang, Z. and Niu, L. (2018). A data model for using OpenStreetMap to integrate indoor and outdoor route planning. Sensors.18:2100.
Weisbrod, G., Vary, D. and Treyz, G. (2003). Measuring the Economic Costs of Urban Traffic Congestion to Business. Journal of the Transportation Research Board. 8:125–139.
WHO. (2016). Ambient Air Pollution: A Global Assessment of Exposure and Burden of Disease. Geneva, Switzerland: WHO.
Yang, Z., & Pun-Cheng, L. S. (2018). Vehicle detection in intelligent transportation systems and its applications under varying environments: A review. Image and Vision Computing, 69, 143-154.
Zaki, J. F., Ali-Eldin, A., Hussein, S. E., Saraya, S. F., & Areed, F. F. (2020). Traffic congestion prediction based on Hidden Markov Models and contrast measure. Ain Shams Engineering Journal, 11(3), 535-551.
Abduljabbar, R., Dia, H., Liyanage, S. and Bagloee, S, A. (2019). Applications of artificial intelligence in transport: An overview. Sustainability. 11: 189; doi:10.3390/su11010189.
Aeberhard, M., Rauch, S., Bahram, M., Tanzmeister, G., Thomas, J., Pilat, Y., Homm, F., Huber, W. and Kaempchen, N. (2015). Experience, results and lessons learned from automated driving on Germany’s highways. IEEE Intell. Transp. Syst. Mag. 7: 42–57.
Aftabuzzaman, M. (2007). Measuring traffic congestion—A critical review. In Proceedings of the 30th Australasian transport research forum (ATRF), Melbourne, Australia.
Anthony, D. (2004). Still Stuck in Traffic: Coping with Peak-Hour Traffic Congestion. Brookings Institution Press Washington, DC.
Azgomi, H.F. and Jamshidi, M. (2018). A brief survey on smart community and smart transportation. In Proceedings of the 2018 IEEE 30th International Conference on Tools with Artificial Intelligence (ICTAI), Volos, Greece, 5–7 November 2018; pp. 932–939.
Biswas, A. and Wang, H. C. (2023). Autonomous vehicles enabled by the integration of IoT, Edge Intelligence, 5G, and Blockchain. Sensors. 23:1963. Available at: https://doi.org/10.3390/ s23041963
BPY, L. and Huang Z. (2022). Spatio-temporal variations of traffic congestion under work from home (WFH) arrangements: Lessons learned from COVID-19. Cities. 2022 May; 124:103610.
Burton, K.J. (2010). Transport economics. Elgar. ed. 3rd.
Chen, Q., & Wang, W. (2019, December). Multi-modal neural network for traffic event detection. In 2019 IEEE 2nd International Conference on Electronics and Communication Engineering (ICECE) (pp. 26-30). IEEE.
Conde, M. L., & Twinn, I. How Artificial Intelligence is Making Transport Safer, Cleaner, More Reliable and Efficient in Emerging Markets. 2019.
Datta, S.K., Da Costa, R.P.F., Härri, J. and Bonnet, C. (2016). Integrating connected vehicles in internet of things ecosystems: Challenges and solutions. In Proceedings of the 2016 IEEE 17th International Symposium on a World of Wireless, Mobile and Multimedia Networks (WoWMoM), Coimbra, Portugal, 21–24 June 2016, pp. 1–6.
de Souza, A. M., & Villas, L. A. (2015). A new solution based on inter-vehicle communication to reduce traffic jam in highway environment. IEEE Latin America Transactions, 13(3), 721-726.
Dikshit, S., Atiq, A., Shahid, M., Dwivedi, V., & Thusu, A. (2023). The Use of Artificial Intelligence to Optimize the Routing of Vehicles and Reduce Traffic Congestion in Urban Areas. EAI Endorsed Transactions on Energy Web, 10.
Djahel, S., Doolan, R. and Muntean G.M. (2015). A communications-oriented perspective on traffic management systems for smart cities: challenges and innovative approaches. IEEE Commun. Surv. Tutor. 17(1): 125–151.
DoT. (2015). Traffic congestion and reliability: trends and advanced strategies for congestion mitigation. Available at:
ESCWA. (2019). SDG 7 IN ARAB REGION. policy Brief. No. 10United Nations.
European Conference of Ministers of Transport. (2007). Cutting transport CO2 emissions: What progress?
Falcocchio, J.C. and Levinson, H.S. (2015). Road traffic congestion: a concise guide (Springer International Publishing AG.
Fattah, M. A., Morshed, S. R. and Kafy, A. A. (2022). Insights into the socio-economic impacts of traffic congestion in the port and industrial areas of Chittagong city, Bangladesh. Transportation Engineering. 9: 100-122.
Guerrero-Ibanez, J.A., Zeadally, S. and Contreras-Castillo, J. (2015). Integration challenges of intelligent transportation systems with connected vehicles, cloud computing, and internet of things technologies. IEEE Wirel. Commun. 22:122–128.
Gomathi, B., Ashwin, G. (2022). Intelligent Traffic Management System Using YOLO Machine Learning Model. In: Peter, J.D., Fernandes, S.L., Alavi, A.H. (eds) Disruptive Technologies for Big Data and Cloud Applications. Lecture Notes in Electrical Engineering, vol 905. Springer, Singapore.
Haque, A.B., Bhushan, B. and Dhiman, G. (2022). Conceptualizing smart city applications: Requirements, architecture, security issues, and emerging trends. Expert Syst. 39: e12753.
Health Effect Institute (HEI) Panel on The Health Effects of Traffic-Related Air Pollution (2010). Traffic-Related Air Pollution: A Critical Review of the Literature on Emissions, Exposure and Health Effects. HEI Special Report 17. Boston, MA: Health Effect Institute.
Herath, H.M.K. and Mittal, M. (2022). Adoption of artificial intelligence in smart cities: A comprehensive review. International Journal of Information Management Data Insights. 2 (1):100076. Available at: HTTPs://doi.org/10.1016/j.jjimei.2022.100076. http://www.ops.fhwa.dot.gov/congestion_report/chapter2.htm
Huang, X., Yang, X., Chang, Y. (2007). Causes of urban traffic congestion. Journal of Transportation Engineering and Information. 5: 108-113.
Hussain, R. and Zeadally, S. (2018). Autonomous cars: Research results, issues, and future challenges. IEEE Commun. Surv. Tutor. 21:1275–1313.
Iyer, L.S. (2021).AI enabled applications towards intelligent transportation. Transportation Engineering. 5:100083 (ISSN 2666-691X). Available at: https://www.sciencedirect.com/science/article/pii/S2666691X21000397 .
Karouani, Y., Elhoussaine, Z. (2018). Toward an Intelligent Traffic Management Based on Big Data for Smart City. In: Ben Ahmed, M., Boudhir, A. (eds) Innovations in Smart Cities and Applications. SCAMS 2017. Lecture Notes in Networks and Systems, vol 37. Springer, Cham.
Kaysi, I., & Chaaban, F. B. (2013). Transitioning to the green economy: the Arab transport sector policy brief. International Journal of Sustainable Development and Planning, 8(3), 305-320.
Kim, D., & Jeong, O. (2019). Cooperative traffic signal control with traffic flow prediction in multi-intersection. Sensors, 20(1), 137.
Kong, X., Xu, Z., Shen, G., Wang, J., Yang, Q., & Zhang, B. (2016). Urban traffic congestion estimation and prediction based on floating car trajectory data. Future Generation Computer Systems, 61, 97-107.
Li, Y. (2021). IF-MABAC method for evaluating the intelligent transportation system with intuitionistic fuzzy information. Journal of Mathematics. Volume 2021 | Article ID 5536751 > Available at: https://doi.org/10.1155/2021/5536751
Lin, C., Han, G., Du, J., Xu, T., Shu, L. and Lv, Z. (2020). Spatiotemporal congestion-aware path planning toward intelligent transportation systems in software-defined smart city IoT. IEEE Internet Things J. 7: 8012–8024.
Litman, T. (2007). Congestion reduction strategies: Identifying and evaluating strategies to reduce traffic congestion; Victoria Transport Policy Institute: Victoria, BC, Canada.
Lopez Conde, M., & Twinn, I. (2019). How artificial intelligence is making transport safer, cleaner, more reliable and efficient in emerging markets.
Mandal, V., Mussah, A. R., Jin, P., & Adu-Gyamfi, Y. (2020). Artificial intelligence-enabled traffic monitoring system. Sustainability, 12(21), 9177.
McGregor, R.V., Eng, P. and MacIver, A. (2003). Regional its architectures—From policy to project implementation. In Proceedings of the Transportation Factor 2003. Annual Conference and Exhibition of the Transportation Association of Canada. (Congres et Exposition Annuels de l’Association des transport du Canada) Transportation Association of Canada (TAC), St. John’s, NL, Canada.
Ministry of Land, Infrastructure, Transport and Tourism 2018: Roads in Japan. MLIT. Tokyo, Japan.
Mohammadi, M. and Al-Fuqaha, A. (2018). Enabling cognitive smart cities using big data and machine learning: Approaches and challenges. IEEE Commun. Mag. 56: 94–101.
Mussone, L., Grant-Muller, S. and Laird, J. (2015). Case Stud. Transp. Policy. 3: 44–54.
Nasim, R. and Kassler, A. (2012). Distributed architectures for intelligent transport systems: A survey. In Proceedings of the 2012 Second Symposium on Network Cloud Computing and Applications, London, UK 3–4 December 2012. pp. 130–136.
Nigam, N., Singh, D.P. and Choudhary, I. (2023). A review of different components of the intelligent traffic management system (ITMS). Symmetry, 15, 583. https:// doi.org/10.3390/sym15030583.
Nikitas, A., Michalakopoulou, K., Njoya, E., T. and Karampatzakis, D. (2020). Artificial intelligence, transport, and the smart city: definitions and dimensions of a new mobility era. Sustainability.12: 2789; doi:10.3390/su12072789.
Oladimeji, D., Gupta, K., Kose, N.A., Gundogan, K., Ge, L. and Liang, F. (2023). Smart Transportation: An overview of technologies and applications. Sensors. 23: 3880. Available at: https://doi.org/10.3390/ s23083880.
Olugbade, S., Ojo, S., Imoize, A. L., Isabona, J., & Alaba, M. O. (2022). A review of artificial intelligence and machine learning for incident detectors in road transport systems. Mathematical and Computational Applications, 27(5), 77.
Ouallane, A. A., Bahnasse, A., Bakali, A., & Talea, M. (2022). Overview of road traffic management solutions based on IoT and AI. Procedia Computer Science, 198, 518-523.
Pan, J., Popa, I.S., Borcea, C. (2016). Divert: a distributed vehicular traffic re-routing system for congestion avoidance. IEEE T Mobile Comput. PP (99): 1.
Pop, M.D., Pandey, J. and Ramasamy, V. (2020). Future networks 2030: Challenges in intelligent transportation systems. In Proceedings of the 2020 8th International Conference on Reliability, Infocom Technologies and Optimization (Trends and Future Directions) (ICRITO), Noida, India, 4–5 June 2020, pp. 898–902.
Reed, T. and Kidd, J. (2019). Global traffic scorecard; INRIX Research: Altrincham, UK.
Rocha Filho, G.P., Meneguette, R.I., Neto, J.R.T., Valejo, A., Weigang, L., Ueyama, J., Pessin G. and Villas, L.A. (2020). Enhancing intelligence in traffic management systems to aid in vehicle traffic congestion problems in smart cities. Ad Hoc Netw. 107:102265.
Sarker, I.H., Kayes, A. and Watters, P. (2019). Effectiveness analysis of machine learning classification models for predicting personalized context-aware smartphone usage. J. Big Data. 6:1–28.
Seo, S. B., & Singh, D. (2018, November). Smart Town Traffic Management System Using LoRa and Machine Learning Mechanism. ReSENSE Lab, Hankuk University of Foreign Studies, Global Campus – Yongin, South Korea.
Shukla, S., Balachandran, K. and Sumitha, V. (2016). A framework for smart transportation using Big Data. In Proceedings of the 2016 International Conference on ICT in Business Industry & Government (ICTBIG), Indore, India, 18–19 November 2016, pp. 1–3.
Sriratnasari, S.R., Wang, G., Kaburuan, E.R. and Jayadi, R. (2019). Integrated smart transportation using IoT at DKI Jakarta. In Proceedings of the 2019 International Conference on Information Management and Technology (ICIMTech), Bali, Denpasar, 19–20 August 2019. Volume 1, pp. 531–536.
Statista. (2023). MENA Road Traffic Delay by City. Retrieved March 4, 2023, from https://www.statista.com/statistics/1331996/mena-road-traffic-delay-by-city/#statisticContainer
Stipancic, J., Miranda-Moreno, L. and Saunier, N. (2017). Impact of congestion and traffic flow on the crash frequency and severity: application of smartphone-collected GPS travel data. Transportation Research Record. 2659(1): 43-54.
Systematics, C. (2005). Traffic congestion and reliability: Trends and advanced strategies for congestion mitigation; Cambridge Systematics Inc.: Cambridge, MA, USA.
Tang, C., Hu, W., Hu, S. and Stettler, M.E.J. (2020). Urban traffic route guidance method with high adaptive learning ability under diverse traffic scenarios. IEEE Trans. Intell. Transp. Syst.22:2956–2968.
Umesh, M. (2022). Improving India’s traffic management using intelligent transportation systems. Electronic Theses, Projects, and Dissertations. Available at: https://scholarworks.lib.csusb.edu/etd/1548.
United Nations, (2023). The State of Arab Cities Report 2022. United Nations Publications.
Wang, Z. and Niu, L. (2018). A data model for using OpenStreetMap to integrate indoor and outdoor route planning. Sensors.18:2100.
Weisbrod, G., Vary, D. and Treyz, G. (2003). Measuring the Economic Costs of Urban Traffic Congestion to Business. Journal of the Transportation Research Board. 8:125–139.
WHO. (2016). Ambient Air Pollution: A Global Assessment of Exposure and Burden of Disease. Geneva, Switzerland: WHO.
Yang, Z., & Pun-Cheng, L. S. (2018). Vehicle detection in intelligent transportation systems and its applications under varying environments: A review. Image and Vision Computing, 69, 143-154.
Zaki, J. F., Ali-Eldin, A., Hussein, S. E., Saraya, S. F., & Areed, F. F. (2020). Traffic congestion prediction based on Hidden Markov Models and contrast measure. Ain Shams Engineering Journal, 11(3), 535-551.
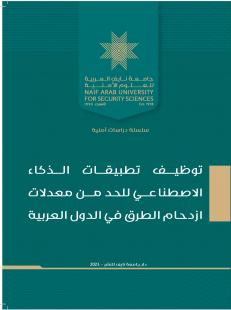